AI-Driven Forecasting: The Key to Long-Term Business Success
Strategic business planning, specifically ‘Strategic Business Planning: Leveraging AI-Driven Forecasting,’ is a crucial procedure that steers a society’s leadership and decision-making for long-term success. Firstly, it concerns establishing plans, choosing the best way to acquire them, and finally, aligning help to make them happen. Artificial intelligence (AI) has emerged as a transformative power in strategic planning in recent years. Consequently, it presents new ways to examine data, forecast trends, and make knowledgeable determinations.
Benefits of AI-Driven Forecasting
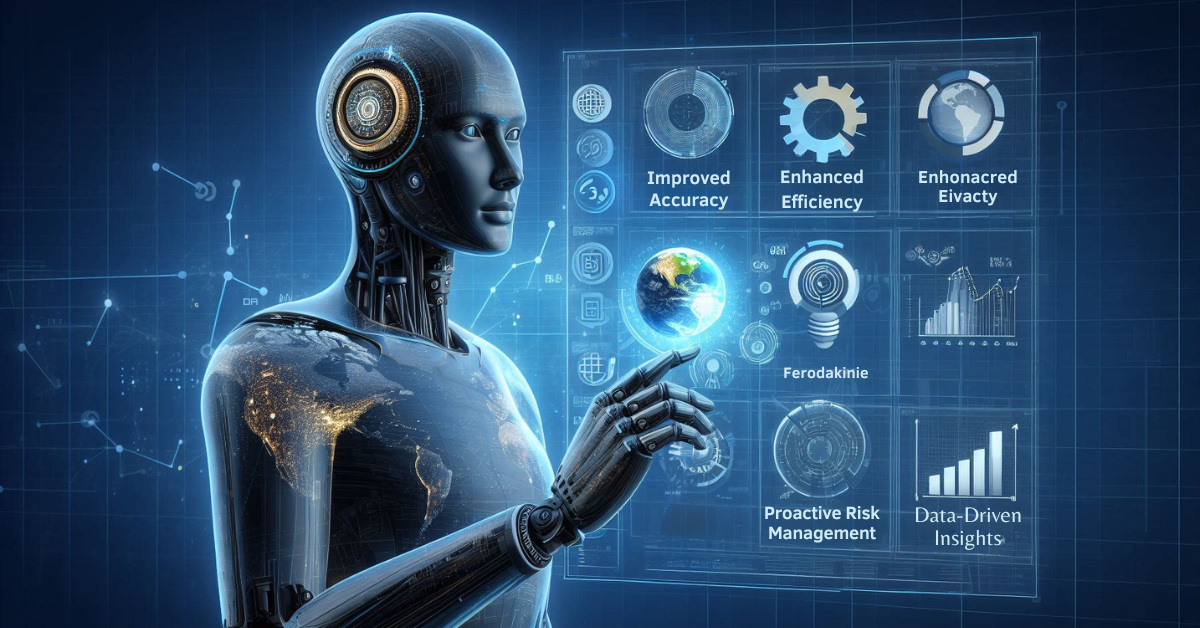
- Improved Accuracy: AI algorithms can examine extensive data, recognizing designs and trends that human reviewers might forget. This leads to more precise forecasts, allowing better-informed strategic judgments.
- Enhanced Efficiency: AI automates repetitious data research and forecasting assignments, letting leaders focus on strategic review and decision-making.
- Proactive Risk Management: AI helps determine possible risks and possibilities earlier, letting leaders mitigate hazards or capitalize on new opportunities proactively.
- Data-Driven Insights: AI declares hidden directions and practices in data, supplying practical insights that report strategic determinations. This discernment reveals new possibilities or highlights regions of concern, enabling leaders to make data-driven decisions aligned with their strategic goals.
We can also read for more details (These tools also constantly rebalance demand and supply by analyzing all available data and constraints.)
Types of AI Forecasting Tools
Technical Deep Dive:
- Machine Learning: At the core of AI forecasting lies machine learning, which provides the algorithms and techniques for AI systems to learn from data and adapt to new conditions. Algorithms learn from historical data to make future predictions. Common types include linear regression, support vector machines, and decision trees.
- Deep Learning: A subset of machine learning, deep learning models handle complex data structures and uncover deeper patterns. They often involve artificial neural networks with multiple layers, allowing them to learn complex relationships between data points.
- Statistical Models: These models use established statistical methods to analyze data and make forecasts. They are often based on assumptions about the underlying distribution of the data and can be effective for specific forecasting problems.
Each tool has strengths and applications, depending on the nature of the data and the organization’s specific forecasting needs.
Read Also: Financial Opportunities: AI-Enhanced Interfaces for a More Equitable Future
Integration with Existing Processes
Data Quality Considerations:
AI forecasting can be incorporated into business planning strategies without disrupting existing workflows. However, ensuring the data provided in AI models is high quality and correctly prepared is essential. This might concern cleaning the data to clear errors or inconsistencies, managing missing values, and ensuring the data represents the forecasted phenomena. Additionally, considering the ethical essence of data supply and usage is essential to ensure data is amassed and used responsibly and complies with relevant regulations.
Case Studies and Examples
Expanded Range of Industries:
Numerous businesses successfully use AI forecasting to improve their strategic planning. These businesses have seen positive impacts such as increased revenue, reduced costs, and improved market share.
- Walmart: Uses AI to forecast demand for over 200,000 items, leading to a 50% reduction in out-of-stock items and a 10% increase in sales.
- Netflix: Uses AI to predict which movies and TV shows its subscribers are most likely to enjoy, resulting in an 80% reduction in customer churn.
- Amazon: Uses AI to predict customer behavior and recommend products, leading to a 35% increase in revenue from cross-selling and upselling.
- HSBC: Uses AI to predict customer creditworthiness, reducing loan defaults by 20%.
- Siemens: Uses AI to predict equipment failures, reducing downtime and maintenance costs by 15%.
Challenges and Limitations
Exploration of Potential Risks:
Despite its possibility, AI-driven forecasting has challenges and restrictions. Firstly, data intolerance can lead to crooked forecasts, especially if the data used to train the AI model is biased. Secondly, the interpretability of AI models can be challenging; it often requires significant effort to comprehend why an AI model made a particular forecast. Consequently, more clarity is needed to make it demanding to trust the model’s predictions and limit its service in decision-making. Additionally, ethical considerations come into play, particularly when AI makes conclusions that affect people’s lives. Thus, it’s essential to ensure that AI is responsible and ethical, bypassing bias, privacy violations, or other dangerous outcomes.
Strategies for mitigating these challenges include:
- Using exemplary AI models when interpretability is critical.
- Securing representative and fair data.
- Specifying ethical approaches for using AI in judgment.
Additionally, it is essential to recognize that AI is a tool and should be utilized by human leadership and decision-making.
Read Also: Worldcoin: A New Dawn for Global Financial Access through AI
The Future of AI-Driven Forecasting
Emerging Trends:
AI-driven forecasting is yet in its earlier growth stages, but it can potentially revolutionize strategic business planning. We want to see even more refined AI models that can regulate more complicated data and make more precise forecasts. We also wish AI forecasting to grow and be more integrated into business planning techniques, helping leaders create real-time data-driven determinations.
Additionally, we will see the emergence of new directions in AI forecasting, like the use of explainable AI to enhance traditional clarity and the integration of AI with other technologies like blockchain and the Internet of Things (IoT) to build stronger and broader forecasting systems. For further detailed read this (AI systems are expected to incorporate more explainable AI techniques and integrate with other emerging technologies like blockchain and edge computing.)
The Impact of AI on Business Leaders
Human & AI Collaboration:
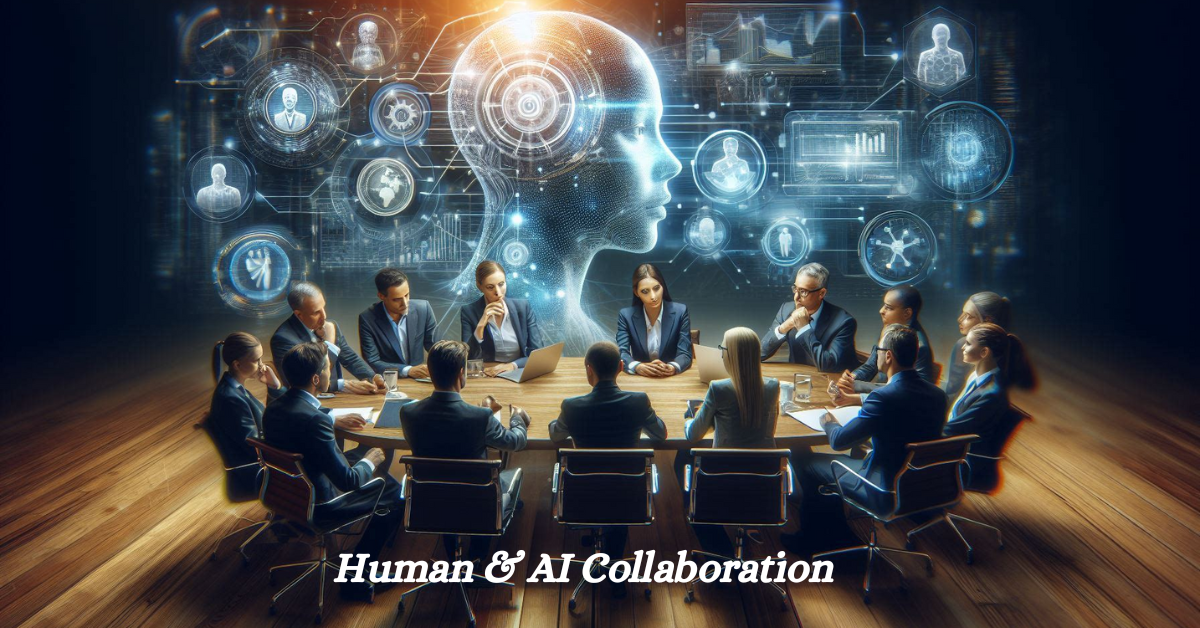
The elevation of AI-driven forecasting will have a notable effect on the role of business executives. Leaders must develop new talents and capacities to work virtually with AI. This possesses a comprehension of the capacities and boundaries of AI. They can interpret AI wisdom and make decisions based on data and human review. Leaders must also design to adjust their communities to the changing landscape of strategic planning, guaranteeing they have the data infrastructure, technology, and procedures to support AI-driven forecasting. However, it’s necessary to remember that AI does not replace human leaders. Instead, AI should seen as an assertive tool that can increase human abilities and enable leaders to make more profitable decisions.
Read Also: AI: The Key to Unlocking Financial Inclusion for 1.7 Billion People
Framework for Assessing AI Readiness
To assess their readiness for AI-driven forecasting, leaders can use the following framework:
- Data readiness: Do they have the data to train and validate AI models?
- Technology readiness: Do they have the technology infrastructure to support AI forecasting?
- Organizational readiness: Do they have the organizational culture and processes to support the adoption of AI?
- Ethical readiness: Do they have ethical guidelines and oversight mechanisms in place to ensure the responsible use of AI?
By working through this framework, leaders can identify areas for improvement to ensure that their organization is ready to leverage AI-driven forecasting for strategic business planning.
Conclusion
Finally, AI-powered forecasting significantly boosts strategic planning through higher accuracy, increased efficiency, and risk management, which is too aggressive and is data-driven wisdom. Nevertheless, it is crucial to realize AI’s possible issues and disadvantages and try to overcome them.
Adopting AI as an assertive tool for strategic planning can enable managers to make knowledgeable decisions and gain long-term success. As with any tool, the key is using it wisely, knowing its strengths and limitations, and meeting with human expertise and determination.